Janet B. Pierrehumbert, University of Oxford
Title: The cognitive status of simple and complex models
Time: Monday, 26 October, 18:00-19:00 (GMT+8)
We are proud to announce that one of the keynote speeches will be delivered by this year's ISCA medalist Janet B. Pierrehumbert.
Abstract
Human languages are extraordinarily rich systems. They have extremely large lexical inventories, and the elements in these inventories can be combined to generate a potentially unbounded set of distinct messages. Regularities at many different levels of representation — from the phonetic level through the syntax and semantics — support people's ability to process mappings between the physical reality of speech, and the objects, events, and relationships that speech refers to. However, human languages also simplify reality. The phonological system establishes equivalence classes amongst articulatory-acoustic events that have considerable variation at the parametric level. The semantic system similarly establishes equivalence classes amongst real-world phenomena having considerable variation.
The tension between simplicity and complexity is a recurring theme of research on language modelling. In this talk, I will present three case studies in which a pioneering simple model omitted important complexities that were either included in later models, or that remain as challenges to this day. The first is the acoustic theory of speech production, as developed by Gunnar Fant, the inaugural Medal recipient in 1989. By approximating the vocal tract as a half-open tube, it showed that the first three formants of vowels (which are the most important for the perception of vowel quality) can be computed as a linear systems problem. The second is the autosegmental-metrical theory of intonation, to which I contributed early in my career. It made the simplifying assumption that the correct model of phonological representation will support the limited set of observed non-local patterns, while excluding non-local patterns that do not naturally occur. The third case concerns how word-formation patterns are generalised in forming new words, whether though inflectional morphology (as in “one wug; two wugs”) or derivational morphology (as in “nickname, unnicknameable”). Several early models of word-formation assume that the morphemes are conceptual categories, sharing formal properties of other categories in the cognitive system.
For all three case studies, I will suggest that — contrary to what one might imagine — the simple models enjoyed good success precisely because they were cognitively realistic. The most successful early models effectively incorporated ways in which the cognitive system simplifies reality. These simplifications are key to the learnability and adaptability of human languages. The simplified core of the system provides the scaffolding for more complex or irregular aspects of language. In progressing from simple models to fully complex models, we should make sure we continue to profit from insights into how humans learn, encode, remember, and produce speech patterns.
Janet B. Pierrehumbert is the Professor of Language Modelling in the Department of Engineering Science at the University of Oxford. She received her BA in Linguistics and Mathematics at Harvard in 1975, and her Ph.D in Linguistics from MIT in 1980. Much of her Ph.D dissertation research on English prosody and intonation was carried out at AT&T Bell Laboratories, where she was also a Member of Technical Staff from 1982 to 1989. At AT&T Bell Labs, she collaborated with 2015 ISCA Medalist Mary Beckman on a theory of tone structure in Japanese, and with 2011 ISCA Medalist Julia Hirschberg on a theory of intonational meaning. After she moved to Northwestern University in1989, her research program used a wide variety of experimental and computational methods to explore how lexical systems emerge in speech communities.
She showed that the mental representations of words are at once abstract and phonetically detailed, and that social factors interact with cognitive factors as lexical patterns are learned, remembered, and generalized. Pierrehumbert joined the faculty at the University of Oxford in 2015 as a member of the interdisciplinary Oxford e-Research Centre; she is also an adjunct faculty member at New Zealand Institute of Language, Brain, and Behaviour. Her current research uses machine-learning methods to model the dynamics of on-line language. She is a founding member of the Association for Laboratory Phonology, and a Fellow of the Linguistic Society of America, the Cognitive Science Society, and the American Academy of Arts and Sciences. She was elected to the National Academy of Sciences in 2019.
Barbara Shinn-Cunningham, Carnegie Mellon University
Title: Brain networks enabling speech perception in everyday settings
Time: Tuesday, 27 October, 18:00-19:00 (GMT+8)
Abstract
While cocktail parties aren't as common as they once were, we all can recall the feeling. You are at a loud party, in a boring conversation. Though you nod politely at all the right moments, your brain is busy listening to the juicy gossip in the interchange behind you. How is it that your brain enables this feat of volitionally directing attention, determining what sound energy is from what sound source, letting through sounds that seem important while filtering out the rest? How is it that unexpected sounds, like the sudden crash of a shattering window, interrupt volitional attention? This talk will explain what we know about control of both spatial and non-spatial processing of sound, based on neuroimaging and behavioral studies, and discuss ways this knowledge can be utilized in developing new assistive listening devices.
Barbara Shinn-Cunningham is an electrical engineer turned neuroscientist who uses behavioral, neuroimaging, and computational methods to understand auditory processing and perception. Her interests span from sensory coding in the cochlea to influences of brain networks on auditory processing in cortex (and everything in between). She is the Cowan Professor of Auditory Neuroscience in and Inaugural Director of the Neuroscience Institute at Carnegie Mellon University, a position she took up after over two decades on the faculty of Boston University. In her copious spare time, she competes in saber fencing and plays the oboe/English horn. She received the 2019 Helmholtz-Rayleigh Interdisciplinary Silver Medal and the 2013 Mentorship Award, both from the Acoustical Society of America (ASA). She is a Fellow of the ASA and of the American Institute for Medical and Biological Engineers, a lifetime National Associate of the National Research Council, and a recipient of fellowships from the Alfred P Sloan Foundation, the Whitaker Foundation, and the Vannevar Bush Fellows program.
Lin-shan Lee, National Taiwan University
Title: Doing Something we Never could with Spoken Language Technologies
-from early days to the era of deep learning
Time: Wednesday, 28 October, 18:00-19:00 (GMT+8)
Abstract
Some research effort tries to do something better, while some tries to do something we never could. Good examples for the former include having aircrafts fly faster, and having images look more beautiful ; while good examples for the latter include developing the Internet to connect everyone over the world, and selecting information out of everything over the Internet with Google ; to name a few. The former is always very good, while the latter is usually challenging.
This talk is about the latter.
A major problem for the latter is those we could never do before was very often very far from realization. This is actually normal for most research work, which could be enjoyed by users only after being realized by industry when the correct time arrived. The only difference is here we may need to wait for longer until the right time comes and the right industry appears. Also, the right industry eventually appeared at the right time may use new generations of technologies very different from the earlier solutions found in research.
In this talk I'll present my personal experiences of doing something we never could with spoken language technologies, from early days to the era of deep learning, including how I considered, what I did and found, and what lessons we can learn today, ranging over various areas of spoken language technologies.
Lin-shan Lee has been teaching in Electrical Engineering and Computer Science at National Taiwan University since 1979.
He invented, published and demonstrated the earliest but very complete set of fundamental technologies and systems for Chinese spoken language technologies including TTS (1984-89), natural language grammar and parser (1986-91) and LVCSR (1987-97), considering the structural features of Chinese language (monosyllable per character, limited number of distinct monosyllables, tones, etc.) and the extremely limited resources.
He then focused his work on speech information retrieval, proposing a whole set of approaches making retrieval performance less dependent on ASR accuracy, and improving retrieval efficiency by better user-content interaction. This part of work applies equally to all different languages, and was described as the stepping stones towards "a spoken version of Google" when Nature selected him in 2018 as one of the 10 "Science Stars of East Asia" in a special issue on scientific research in East Asia.
Shehzad Mevawalla , Amazon Alexa
Title: Successes, Challenges and Opportunities for Speech Technology in Conversational Agents
Time: Thursday, 29 October, 18:00-19:00 (GMT+8)
Abstract
From the early days of modern ASR research in the 1990s, one of the driving visions of the field has been a computer-based assistant that could accomplish tasks for the user, simply by being spoken to. Today, we are close to achieving that vision, with a whole array of speech-enabled AI agents eager to help users. Amazon’s Alexa pioneered the AI assistant concept for smart speaker devices enabled by far-field ASR. It currently supports billions of customer interactions per week, on over 100 million devices across multiple languages. This keynote will give an overview of the interplay between underlying speech technologies, including wakeword detection, endpointing, speaker identification, and speech recognition that enable Alexa. We highlight the complexities of combining these technologies into a seamless and robust speech-enabled user experience under large production load and real-time constraints. Interesting algorithmic and engineering challenges arise from choices between deployment in the cloud versus on edge devices, and from constraints on latency and memory versus trade-offs in accuracy. Adapting recognition systems to trending topics, changing domain knowledge bases, and to the customer’s personal catalogs adds additional complexity, as does the need to support adaptive conversational behavior (such as normal versus whispered speech). We also dive into the unique data aspects of large-scale deployments like Alexa, where a continuous stream of unlabeled data enables successful applications of weakly supervised learning. Finally, we highlight problems for the speech research community that remain to be solved before the promise of a fully natural, conversational assistant is fully realized.
Shehzad Mevawalla is a Director in Amazon and responsible for automatic speech recognition, speaker recognition and paralinguistics in Alexa world-wide. Recognition from far-field speech input is a key enabling technology for Alexa, and Shehzad and his team work to advance the state of the art in this area for both cloud and edge device. A thirteen-year veteran at Amazon, he has held a variety of senior technical roles, which include supply chain optimization, marketplace trust and safety, and business intelligence, prior to his position with Alexa. Before joining Amazon in 2007, Shehzad was Director of Software at HNC, a company that specialized in financial AI, where he worked on products that used neural networks to detect fraud. Shehzad holds a Master’s degree in Computer Engineering and a Bachelor’s degree in Computer Science, both from the University of Southern California.
Janet B. Pierrehumbert, University of Oxford
Title: The cognitive status of simple and complex models
Time:Monday, 26 October, 18:00-19:00 (GMT+8)
We are proud to announce that one of the keynote speeches will be delivered by this year's ISCA medalist Janet B. Pierrehumbert.
Abstract
Human languages are extraordinarily rich systems. They have extremely large lexical inventories, and the elements in these inventories can be combined to generate a potentially unbounded set of distinct messages. Regularities at many different levels of representation — from the phonetic level through the syntax and semantics — support people's ability to process mappings between the physical reality of speech, and the objects, events, and relationships that speech refers to. However, human languages also simplify reality. The phonological system establishes equivalence classes amongst articulatory-acoustic events that have considerable variation at the parametric level. The semantic system similarly establishes equivalence classes amongst real-world phenomena having considerable variation.
The tension between simplicity and complexity is a recurring theme of research on language modelling. In this talk, I will present three case studies in which a pioneering simple model omitted important complexities that were either included in later models, or that remain as challenges to this day. The first is the acoustic theory of speech production, as developed by Gunnar Fant, the inaugural Medal recipient in 1989. By approximating the vocal tract as a half-open tube, it showed that the first three formants of vowels (which are the most important for the perception of vowel quality) can be computed as a linear systems problem. The second is the autosegmental-metrical theory of intonation, to which I contributed early in my career. It made the simplifying assumption that the correct model of phonological representation will support the limited set of observed non-local patterns, while excluding non-local patterns that do not naturally occur. The third case concerns how word-formation patterns are generalised in forming new words, whether though inflectional morphology (as in “one wug; two wugs”) or derivational morphology (as in “nickname, unnicknameable”). Several early models of word-formation assume that the morphemes are conceptual categories, sharing formal properties of other categories in the cognitive system.
For all three case studies, I will suggest that — contrary to what one might imagine — the simple models enjoyed good success precisely because they were cognitively realistic. The most successful early models effectively incorporated ways in which the cognitive system simplifies reality. These simplifications are key to the learnability and adaptability of human languages. The simplified core of the system provides the scaffolding for more complex or irregular aspects of language. In progressing from simple models to fully complex models, we should make sure we continue to profit from insights into how humans learn, encode, remember, and produce speech patterns.
Janet B. Pierrehumbert is the Professor of Language Modelling in the Department of Engineering Science at the University of Oxford. She received her BA in Linguistics and Mathematics at Harvard in 1975, and her Ph.D in Linguistics from MIT in 1980. Much of her Ph.D dissertation research on English prosody and intonation was carried out at AT&T Bell Laboratories, where she was also a Member of Technical Staff from 1982 to 1989. At AT&T Bell Labs, she collaborated with 2015 ISCA Medalist Mary Beckman on a theory of tone structure in Japanese, and with 2011 ISCA Medalist Julia Hirschberg on a theory of intonational meaning. After she moved to Northwestern University in1989, her research program used a wide variety of experimental and computational methods to explore how lexical systems emerge in speech communities.
She showed that the mental representations of words are at once abstract and phonetically detailed, and that social factors interact with cognitive factors as lexical patterns are learned, remembered, and generalized. Pierrehumbert joined the faculty at the University of Oxford in 2015 as a member of the interdisciplinary Oxford e-Research Centre; she is also an adjunct faculty member at New Zealand Institute of Language, Brain, and Behaviour. Her current research uses machine-learning methods to model the dynamics of on-line language. She is a founding member of the Association for Laboratory Phonology, and a Fellow of the Linguistic Society of America, the Cognitive Science Society, and the American Academy of Arts and Sciences. She was elected to the National Academy of Sciences in 2019.
Barbara Shinn-Cunningham, Carnegie Mellon University
Title: Brain networks enabling speech perception in everyday settings
Time: Tuesday, 27 October, 18:00-19:00 (GMT+8)
Abstract
While cocktail parties aren't as common as they once were, we all can recall the feeling. You are at a loud party, in a boring conversation. Though you nod politely at all the right moments, your brain is busy listening to the juicy gossip in the interchange behind you. How is it that your brain enables this feat of volitionally directing attention, determining what sound energy is from what sound source, letting through sounds that seem important while filtering out the rest? How is it that unexpected sounds, like the sudden crash of a shattering window, interrupt volitional attention? This talk will explain what we know about control of both spatial and non-spatial processing of sound, based on neuroimaging and behavioral studies, and discuss ways this knowledge can be utilized in developing new assistive listening devices.
Barbara Shinn-Cunningham is an electrical engineer turned neuroscientist who uses behavioral, neuroimaging, and computational methods to understand auditory processing and perception. Her interests span from sensory coding in the cochlea to influences of brain networks on auditory processing in cortex (and everything in between). She is the Cowan Professor of Auditory Neuroscience in and Inaugural Director of the Neuroscience Institute at Carnegie Mellon University, a position she took up after over two decades on the faculty of Boston University. In her copious spare time, she competes in saber fencing and plays the oboe/English horn. She received the 2019 Helmholtz-Rayleigh Interdisciplinary Silver Medal and the 2013 Mentorship Award, both from the Acoustical Society of America (ASA). She is a Fellow of the ASA and of the American Institute for Medical and Biological Engineers, a lifetime National Associate of the National Research Council, and a recipient of fellowships from the Alfred P Sloan Foundation, the Whitaker Foundation, and the Vannevar Bush Fellows program.
Lin-shan Lee, National Taiwan University
Title: Doing Something we Never could with Spoken Language Technologies
-from early days to the era of deep learning
Time: Wednesday, 28 October, 18:00-19:00 (GMT+8)
Abstract
Some research effort tries to do something better, while some tries to do something we never could. Good examples for the former include having aircrafts fly faster, and having images look more beautiful ; while good examples for the latter include developing the Internet to connect everyone over the world, and selecting information out of everything over the Internet with Google ; to name a few. The former is always very good, while the latter is usually challenging.
This talk is about the latter.
A major problem for the latter is those we could never do before was very often very far from realization. This is actually normal for most research work, which could be enjoyed by users only after being realized by industry when the correct time arrived. The only difference is here we may need to wait for longer until the right time comes and the right industry appears. Also, the right industry eventually appeared at the right time may use new generations of technologies very different from the earlier solutions found in research.
In this talk I'll present my personal experiences of doing something we never could with spoken language technologies, from early days to the era of deep learning, including how I considered, what I did and found, and what lessons we can learn today, ranging over various areas of spoken language technologies.
Lin-shan Lee has been teaching in Electrical Engineering and Computer Science at National Taiwan University since 1979.
He invented, published and demonstrated the earliest but very complete set of fundamental technologies and systems for Chinese spoken language technologies including TTS (1984-89), natural language grammar and parser (1986-91) and LVCSR (1987-97), considering the structural features of Chinese language (monosyllable per character, limited number of distinct monosyllables, tones, etc.) and the extremely limited resources.
He then focused his work on speech information retrieval, proposing a whole set of approaches making retrieval performance less dependent on ASR accuracy, and improving retrieval efficiency by better user-content interaction. This part of work applies equally to all different languages, and was described as the stepping stones towards "a spoken version of Google" when Nature selected him in 2018 as one of the 10 "Science Stars of East Asia" in a special issue on scientific research in East Asia.
Shehzad Mevawalla , Amazon Alexa
Title: Successes, Challenges and Opportunities for Speech Technology in Conversational Agents
Time: Thursday, 29 October, 18:00-19:00 (GMT+8)
Abstract
From the early days of modern ASR research in the 1990s, one of the driving visions of the field has been a computer-based assistant that could accomplish tasks for the user, simply by being spoken to. Today, we are close to achieving that vision, with a whole array of speech-enabled AI agents eager to help users. Amazon’s Alexa pioneered the AI assistant concept for smart speaker devices enabled by far-field ASR. It currently supports billions of customer interactions per week, on over 100 million devices across multiple languages. This keynote will give an overview of the interplay between underlying speech technologies, including wakeword detection, endpointing, speaker identification, and speech recognition that enable Alexa. We highlight the complexities of combining these technologies into a seamless and robust speech-enabled user experience under large production load and real-time constraints. Interesting algorithmic and engineering challenges arise from choices between deployment in the cloud versus on edge devices, and from constraints on latency and memory versus trade-offs in accuracy. Adapting recognition systems to trending topics, changing domain knowledge bases, and to the customer’s personal catalogs adds additional complexity, as does the need to support adaptive conversational behavior (such as normal versus whispered speech). We also dive into the unique data aspects of large-scale deployments like Alexa, where a continuous stream of unlabeled data enables successful applications of weakly supervised learning. Finally, we highlight problems for the speech research community that remain to be solved before the promise of a fully natural, conversational assistant is fully realized.
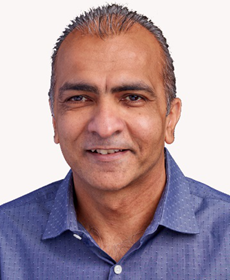
Shehzad Mevawalla is a Director in Amazon and responsible for automatic speech recognition, speaker recognition and paralinguistics in Alexa world-wide. Recognition from far-field speech input is a key enabling technology for Alexa, and Shehzad and his team work to advance the state of the art in this area for both cloud and edge device. A thirteen-year veteran at Amazon, he has held a variety of senior technical roles, which include supply chain optimization, marketplace trust and safety, and business intelligence, prior to his position with Alexa. Before joining Amazon in 2007, Shehzad was Director of Software at HNC, a company that specialized in financial AI, where he worked on products that used neural networks to detect fraud. Shehzad holds a Master’s degree in Computer Engineering and a Bachelor’s degree in Computer Science, both from the University of Southern California.